Introduction
Organizations can gain significant financial advantages by implementing machine learning and other AI technologies for risk management and security-related use cases. Numerous AI-enabled risk management solutions rely on the cloud’s mass computing capacity, which allows for the quick analysis and processing of massive amounts of data. On-premises systems, however, can also facilitate the use of AI in risk management programs. Artificial intelligence risk management empowers organizations to proactively identify and mitigate potential threats by leveraging advanced algorithms and predictive analytics. The development and implementation of an artificial intelligence risk management framework are crucial for ensuring the responsible and secure deployment of advanced technologies in various industries.
Security automation workflows and cybersecurity threat detection initiatives can both benefit from the integration of risk management solutions with AI capabilities. They can also assist corporate executives, risk managers, and security chiefs in making deft decisions in various situations, during incidents, and as part of the business continuity planning process. Text and other unstructured data analysis is a special application for artificial intelligence (AI) technology, such as software for natural language processing.
Applications of AI in Risk Management
AI risk management solutions offer businesses a sophisticated and efficient approach to identifying, analyzing, and mitigating potential risks in real time, enhancing overall strategic decision-making processes. By combining AI risk management and AI-powered CRM, businesses can not only optimize customer interactions and relationships through intelligent insights but also proactively identify and address potential risks, fostering a more secure and efficient operational environment. The following are some of the most typical use cases for AI in support of risk management and mitigation procedures:
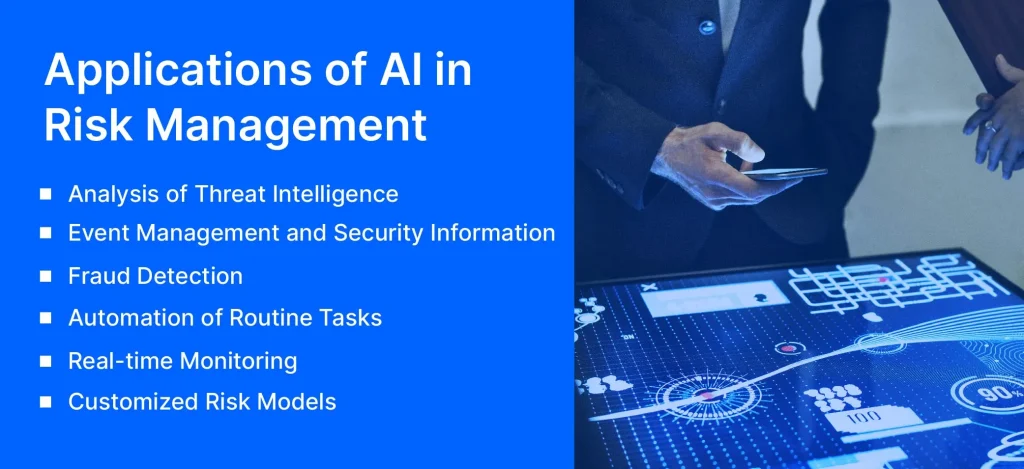
1. Analysis of Threat Intelligence
Threat intelligence data gives businesses insight into things like attacker origins, signs of compromise, usage patterns associated with cloud accounts, and assaults on different kinds of cloud services. Machine learning engines can be used to gather, evaluate, and process threat information streams at scale for risk forecasting models and likelihood estimations. With ransomware infections and cloud account hijacking attacks on the rise, security teams may find that quicker data analysis and predictive intelligence are crucial tools for risk management.
2. Event Management and Security Information
Massive amounts of log data and other recordings of security occurrences are being generated. Security teams must be able to swiftly identify certain threat indicators, identify patterns in events as they happen, and identify events occurring in both cloud and on-premises settings in order to mitigate cybersecurity risks. Machine learning and artificial intelligence (AI) can enhance huge event data processing technology in SIEM procedures to provide more sophisticated alerting and detection capabilities. One cloud-based SIEM solution with AI and machine learning capabilities is Microsoft Sentinel. Implementing a robust risk management AI system enhances organizational resilience by proactively identifying, analyzing, and mitigating potential threats, thereby fostering a more secure and adaptive business environment.
3. Fraud Detection
Fraud detection using machine learning for insurance and financial services companies necessitates a vast array of data types and inputs, along with extensive processing. By supporting text mining, database searches, social network analysis, anomaly detection approaches, and predictive model coupling at scale, artificial intelligence (AI) systems and machine learning engines can aid in the detection of fraudulent transactions and activities. This might also apply to instances of dishonest use of cloud services, including phishing attacks launched via compromised Microsoft 365 accounts.
4. Automation of Routine Tasks
AI streamlines routine risk management tasks, freeing up human resources for more strategic decision-making. Automation reduces the likelihood of errors in data processing and allows risk managers to focus on high-level analyses and strategic planning.
5. Real-time Monitoring
AI systems enable real-time monitoring of risks, providing organizations with instantaneous insights into potential threats. This allows for swift responses and the ability to adapt strategies promptly, minimizing the impact of adverse events.
6. Customized Risk Models
AI allows for the development of highly customized risk models based on an organization’s specific needs and industry dynamics. These tailored models can provide more accurate risk assessments, aligning risk management strategies with the organization’s unique risk profile.
Challenges in Implementing AI in Risk Management
The foundation of any AI system is the data it is trained on. In risk management, historical data is crucial for training algorithms to predict and assess potential risks. However, if the data used is of poor quality or contains inherent biases, it can lead to inaccurate risk assessments.
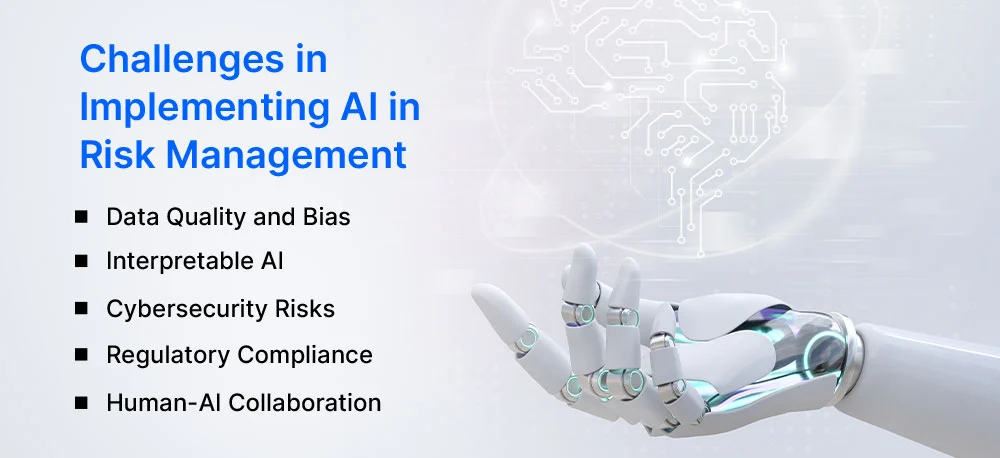
Data Quality and Bias
The effectiveness of AI models is heavily dependent on the quality and diversity of the data they are trained on. Biases present in historical data can perpetuate AI algorithms, leading to skewed risk assessments. Ensuring data accuracy and addressing biases are ongoing challenges in the implementation of AI in risk management.
Interpretable AI
AI models, particularly complex ones like deep neural networks, often operate as “black boxes,” making it challenging for humans to understand the reasoning behind specific decisions. Building interpretable AI models is crucial for risk managers to trust and validate the outcomes.
Cybersecurity Risks
As AI becomes more prevalent in risk management, it also becomes a target for malicious actors. The risk of cyberattacks targeting AI systems introduces new challenges in securing sensitive data and ensuring the integrity of risk management processes.
Regulatory Compliance
The regulatory landscape is often slow to adapt to technological advancements. Implementing AI in risk management requires organizations to navigate complex regulatory frameworks, ensuring compliance while harnessing the benefits of AI tools.
Human-AI Collaboration
The integration of AI in risk management should be viewed as a collaboration between machines and humans. Ensuring effective communication and understanding between AI systems and human decision-makers is crucial to harnessing the full potential of AI in risk management.
Conclusion
Artificial Intelligence has undoubtedly transformed the landscape of risk management, offering unprecedented opportunities to enhance decision making using AI processes and mitigate potential threats. However, organizations must tread carefully, addressing challenges related to data quality, interpretability, cybersecurity, regulatory compliance, and the delicate balance between human and AI collaboration. Many businesses are leveraging AI chatbot development services to enhance customer interactions, streamline communication processes, and provide efficient, round-the-clock support.
Despite the problems raised above, AI will probably become more and more important in corporate risk management initiatives in the future. Risk management teams will continue to gain from the quick analytics processing of big data sets provided by cloud-based AI and machine learning services, which will eliminate many of the drawbacks of the earlier, more labor-intensive risk analysis and risk management procedures. By helping with tasks like data monitoring, risk assessment, and stakeholder communication, generative AI systems may potentially support risk management projects.
Enhance Risk Management with our AI Chatbot Development Services Today!
Follow IntellicoWorks for more insights!